The Problem
As metabolomics took off in the early 2000s, it became increasingly clear that GC-MS data was hampered by its 1950s-era electron ionization – using a single designated ionization energy with the need for derivatization – and a focus on molecules that are stable enough to survive the GC oven. An alternative was needed – one that could harness the emerging power of MS/MS techniques.
Background
For decades, GC-MS was the dominant metabolite and small molecule identification technology, despite its drawbacks. This dominance was primarily due to the impressive size of its chemical libraries; for example, NIST’s library of GC-MS mass spectra, which contained information for over 270,000 individual compounds.
The 2002 Nobel prizes celebrated developments in the now ubiquitous electrospray ionization (ESI). ESI allows for the observation of a broader range of molecules due to its non-destructive nature. Yet, though these newer ESI tandem MS approaches were adopted quickly in metabolomics and proteomics, they were not universally adopted in studies of metabolites and chemical entities because no comprehensive tandem MS databases existed. That is, until a series of three papers (1)(2)(3) documenting breakthroughs using METLIN (a cloud-based and freely available ESI tandem MS library) found themselves challenging the dominance of GC-MS.
The Solution
METLIN had humble beginnings back in 2002 – tens of molecules were slowly acquired if and when standards became available. As you can imagine, the tandem MS data was accumulated at a glacial pace. Skip forward to February 2019: METLIN bypassed the NIST GC-MS database mark with tandem MS fragmentation data for 300,000 molecular standards. In August 2019, it reached the milestone of 500,000 standards (Figure 1), encompassing vast metabolic and chemical diversity (Figure 2). There are experimental data for each molecule in both positive and negative ionization modes, each generated at four different collision energies. Originally designed to facilitate the field of metabolomics, METLIN has now leapfrogged into the broader field of small molecule chemical analysis, including organic chemistry, pharmaceuticals, toxicology, exposure research, and drugs of abuse.
The feat was made possible by a group of highly talented Scripps Research staff with innovative ideas and the drive to see them through. H. Paul Benton and Aries Aisporna combined their efforts to address the critical informatic challenges, which included transferring the standards’ physical information to the MS instrumentation, as well as automating the identity (and data) transfer to METLIN, and – most importantly – automated data curation. Elizabeth Billings, Emily Chen and Winnie Heim designed a preparation approach that maximizes sample transfer and ESI tandem MS data acquisition. Winnie has also played a key role in the collection of retention time and tandem MS data, and manually curating compound data that did not pass the automated curation step – not a trivial endeavor at this scale.
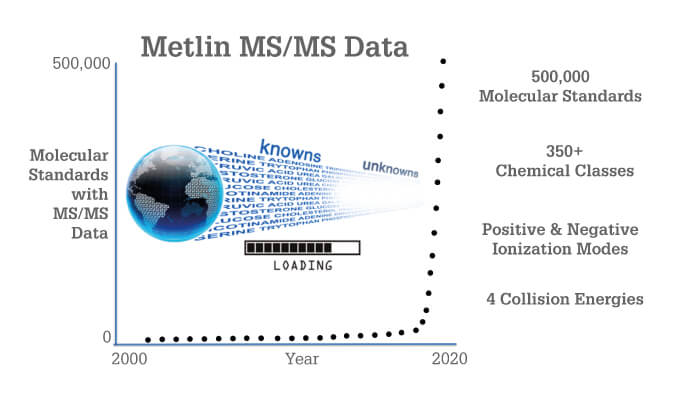
With a success rate of approximately 80 percent, the platform is robust – but it is far from perfect, with around 20 percent of molecules not providing sufficient precursor ionization or suffering isolation window contamination, among other problems. To reach 500,000, we’ve had to analyze over 600,000 molecular standards (at the time of writing), with over 100,000 molecules not passing our automated and manual vetting.
Central to the integrity of any library is the use of standards. As we know all too well, the wrong identification can send our collaborators off on a “wild goose chase” for months – if not years. And though the size of the library is important, the dominant factor in moving ESI tandem MS identification forward is access to standards (just as in GC-MS data). METLIN is projected to grow its tandem MS database to over a million validated molecular standards in 2020, allowing the community to finally move out of the 1950s. Given the obvious benefits of metabolite and chemical entity identification, and the possibility for unknown identification through H. Paul Benton’s original similarity searching (4)(5), METLIN represents an overdue transition to the 21st century that – when complementing GC-MS – is allowing small molecule identification to become significantly more comprehensive.
Beyond the solution
METLIN’s growth will have far-reaching implications, firstly by increasing the ease and reliability of molecular identification exercises, but also by providing researchers with countless further opportunities to exploit the housed data. It is worth noting that METLIN is 30 times bigger than alternative standards databases and is a refined resource that has been widely used for over a decade. It’s certainly come a long way since 2002… But we aren’t finished yet! A number of further developments are planned, including:
• the development of similarity searching for unknown identification (1)(4),
• use of METLIN’s retention time data to facilitate machine learning predictive algorithms,
• introduction of hydrophobicity filtering from retention time data to improve molecular identification,
• molecular structure determination from MS/MS data by machine learning approaches,
• automated generation of multiple reaction monitoring parameters for quantitative analysis (6),
• endogenous and exogenous activity annotations (5),
• and MS/MS-based pathway mapping (7).
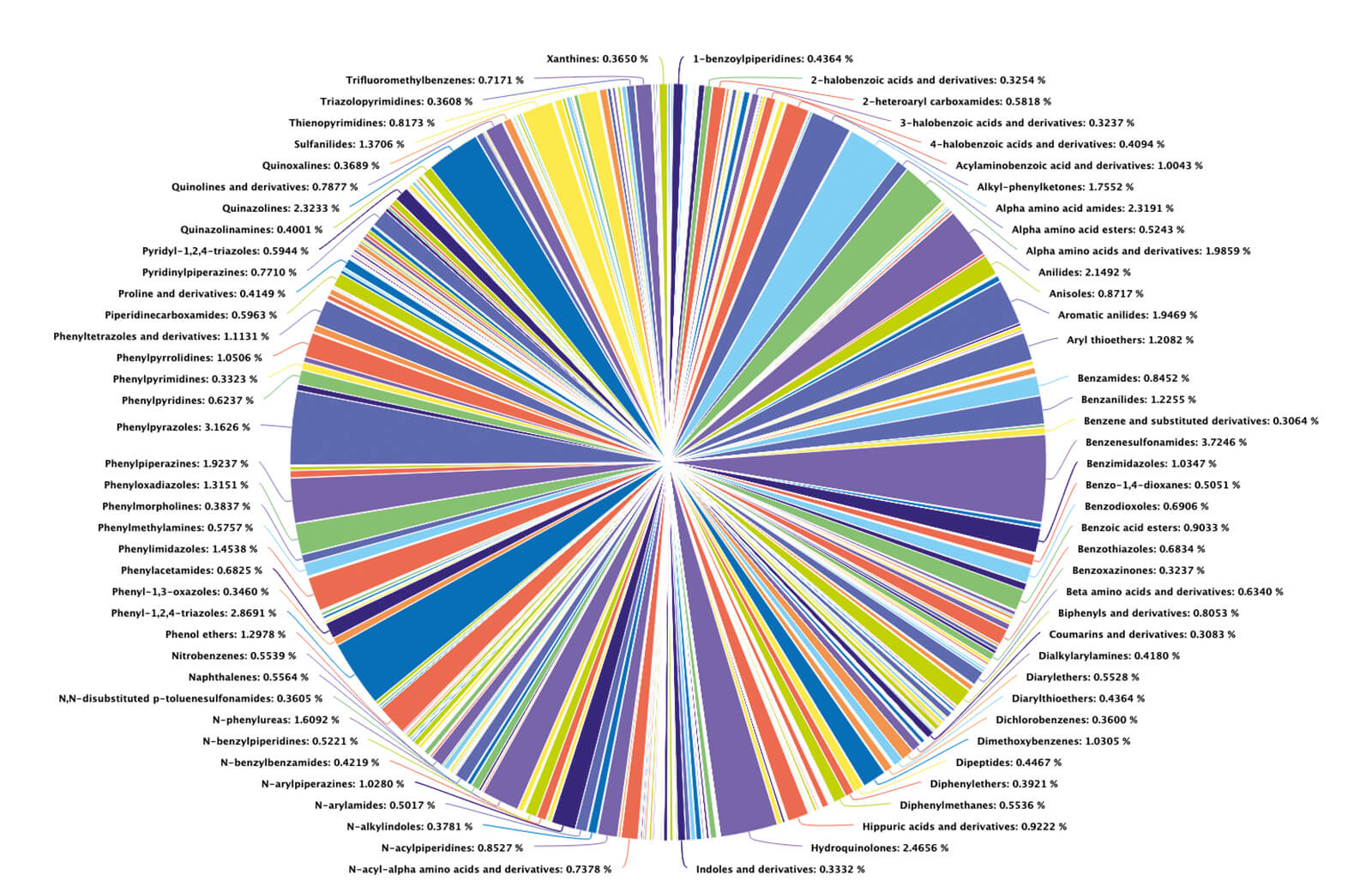
References
- C Guijas et al., “METLIN: a technology platform for identifying knowns and unknowns”, Anal Chem, 90, 3156 (2018). DOI: 10.1021/acs.analchem.7b04424
- MM Rinschen et al., “Identification of bioactive metabolites using activity metabolomics”, Nat Rev Mol Cell Bol, 20, 353 (2019). DOI: 10.1038/s41580-019-0108-4
- X Domingo-Almenara et al., “Autonomous METLIN-guided in-source fragment annotation for untargeted metabolomics”, Anal Chem, 91, 3246 (2019). DOI: 10.1021/acs.analchem.8b03126
- HP Benton et al., “XCMS2: processing tandem mass spectrometry data for metabolite identification and structural characterization”, Anal Chem, 80, 6382 (2008). DOI: 10.1021/ac800795f
- X Domingo-Almenara et al., “Annotation: a computational solution for streamlining metabolomics analysis”, 90, 480 (2018). DOI: 10.1021/acs.analchem.7b03929
- X Domingo-Almenara et al., “XCMS-MRM and METLIN-MRM: a cloud library and public resource for targeted analysis of small molecules”, Nat Methods, 15, 681 (2018). DOI: 10.1038/s41592-018-0110-3
- T Huan et al., “Systems biology guided by XCMS online metabolomics”, Nat Methods, 14. 461 (2017). DOI: 10.1038/nmeth.4260